Interview with Yann Ferguson: going beyond workers’ fears regarding artificial intelligence
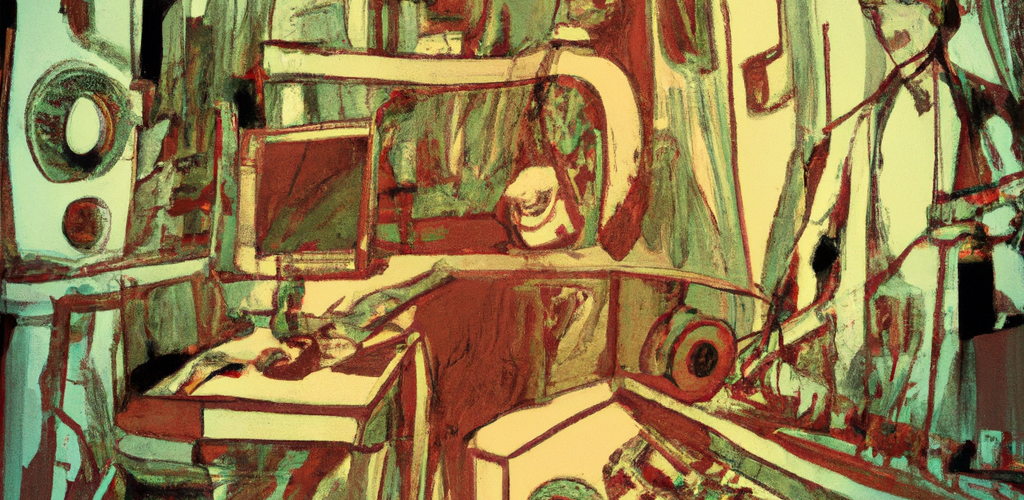
Article author :
Automation, the replacement of work, an increase in productivity, etc. The artificial intelligence (AI) technology boom is said to herald the end of work. But, according to Yann Ferguson, a Doctor in sociology and a specialist on the impact of AI on the job market, a more nuanced look at the issue is warranted.
What is the source of our fears regarding AI?
Yann Ferguson: To answer this question, we have to go back in time to the first applications of AI. The year 1956 is considered the starting point for AI as a distinct research domain. In that year, a major event called the Dartmouth Conference brought together a group of researchers working on computing and cognitive sciences and which wished to explore the possibility of creating an ‘intelligent machine’. Herbert Simon, a Nobel Prize laureate in economics in 1978, was already claiming in 1958 that ‘machines would rapidly be able to do what human beings can do’. Having a Nobel Prize did not prevent him from getting his forecasts wrong. A certain scientific and technological optimism thus led people to overestimate technological power versus the actual intelligence enacted by workers in the workplace. The organisational and Taylorist viewpoint which prevailed at that time meant that work was considered as a simple sequence of instructions given to human beings. To replicate work, all that was needed was to assemble these instructions and to implement them in a computer program. There is a double epistemological error in play here: human thought processes cannot be broken down so easily, and nor can work, either.
In the 1960s, more effort was put into devise computer programs and AI started to be developed, but there was still a lack of computing power, of logic. The world of work was little studied, as was the actual intelligence put to use by workers. In the 1990s, another period when AI gained momentum, expert systems were created. The idea was less to design machines which would be the equivalent of human intelligence, but instead to replace certain tasks or to complete human input. But from a nonetheless very vertical perspective. A subject-matter expert revealed all the secrets and the lines of reasoning they mobilise in their profession to a knowledge engineer, who then converted them into computer language. The engineer programmed the machine in relation to situations imagined beforehand by the expert. This 1990s boom faltered on this occasion not because of a lack of computing power, as progress had been made in this respect, but because of another paradox notably brought to light by Michael Polanyi. This Hungarian polymath and epistemologist established that we know a great deal more than we are capable of expressing. The subject-matter expert we question in regard to their profession is so little aware of their own knowledge that it is impossible for them to express enough information to have any hopes of having their intelligence matched by a machine. And what’s more, the greater our level of expertise is, the more this expertise is implanted in the unutterable of experience at work. This observation strongly limited the relevance of the expert systems developed in this period. Once again, we became aware of the limits to our ability to understand the experience of work, but also of the fact that we were continuing to underestimate the knowledge and know-how of the experts.
If we are today thinking that’s it, we have managed to create machines which match human work, it is because we have changed our approach to AI. AI systems make use of very large quantities of data and create rules on the basis of these tons of data. When the correlations between all these data are robust enough, the machine utilises these correlations like rules in order to solve problems. Some people today feel that AI will be able to create its own character, its own experience, but that is not the case. It will always start from experience to go towards theory.
What scares every worker is the word ‘intelligence’. People consider AI a rival. If we go back to the early days of machine intelligence, before it was even called ‘AI’, the Turing test in 1950 already consisted of saying that if a machine can pass itself off as a human being, we must therefore consider it intelligent. From the outset the mathematician Alan Turing placed this hot topic on the table: in short, if a machine appears intelligent, it is thus the equivalent of human intelligence. This form of non-living intelligence, quite active in the mathematical domains, has capabilities and a power which are stronger than us, without a shadow of a doubt. We fear the discovery of an intelligence superior to us which, if science fiction is to be believed, could develop free will and begin to make decisions in our place. It is a metaphysical fear. Since the early days of computing, we have seen the machine as a reflection of ourselves. Surprising, since it is human beings who create the machines.
What is also feeding the fear in the face of the vast influx of AI in the workplace is that these enormous data systems and the ways in which AI arrives at a result remain opaque. Without being able to understand these machines’ mode of reasoning, the tension between power and opacity remains worrying for the worker. In a company, the injunction to use these intelligent machines can be paradoxical: you have to be capable of using AI whilst remaining responsible for its results.
Many workers consider the use of AI as a time saver. Are we inevitably risking bringing about our downfall in considering these new technologies for the productivity gains they may potentially give rise to?
Y. F.: Historically, we have always considered the machine as a productivity gain in the world of work. In the Marxist view of labour, we set the machine against the human being. It was postulated that in replacing workers with machines, the productivity gains would be astronomical. From a more humanist perspective, however, it is considered that the machine can automate certain tasks which are beneath the human being, so that they can attend to other tasks. That is also the discourse which today frames the debate over AI. The momentum AI is gaining at times wipes out the limits of these technologies, which should push us to think in different ways. In fact, AI is empirical, but it is not a question of the same empirical knowledge as that of a human being. We generalise situations on the basis of very little information and compensate for our gaps with our sensitive knowledge of the world. But, unlike the machine, a human being is bad when it receives too much information. The machine, for its part, is not effective with a little information, but with a lot of data it becomes very effective. The machine is deterministic, predictable, but not very flexible and has no sensitive experience of the world around it, unlike a worker, who is pretty unpredictable but extremely flexible. An asset which is virtually irreplaceable by a machine. They are two very different empirical resources. Two empirical knowledges which we should accentuate in collaboration, not in opposition. The current issue is understanding how to get the two empirical knowledges to interact. Without being able to theorise anything, my research in this area all the same allows a body of evidence to be suggested as to the nature of the machinic revolution we are witnessing. AI will not mean an increase in productivity through the automation of tasks, but instead an increase in quality by means of better interaction between humans and machines. Through positive externality, we may see gains in time and productivity, certainly. But that should not be the objective aimed for from the outset.
Do you have any innovative examples from the various trades which have integrated AIs into their daily routine at work?
Y. F.: I have studied the case of the recruitment profession and the integration of a recruitment assistance system based on an AI in a major public scientific research organisation. They have invested in this programme by making it compulsory for human resources managers. It is a tool which has the job applicants take three tests: a psychometric test, a personality test, as well as a third motivation and reasoning test. The AI analyses the data of each test and the interaction between these data, and correlates them with positions which correspond to the applicant. The developer of this system recommends that its clients use threshold effects: starting from an 80% match, they must absolutely have the applicant take a second job interview; between 80% and 50%, there is some room for discussion; below 50%, it’s pointless. This organisation has decided to make the tool compulsory but does not believe in threshold effects, and has therefore asked the recruiters to use this system as they wish. In their recruitment sequence, the recruiter therefore retains their free will and may have the applicants first take the tests before the interview, or do it the other way round. Without being obliged to look at the results before the interview.
Where are we in terms of AI being accepted at the workplace?
Y. F.: One of the big questions which gets asked about the application of AI within a company, without taking into account solely generative AI, is why the deployment rate of its applications is still so low. The major companies have carried out trials, but over 90% of the AI systems which could become new norms if we produced them have still not been deployed. The reasons are simple: the AI systems are not considered functional, even if most of the time they achieve good key performance indicators (Editor’s note, the KPIs). Consequently, one might wonder why these experiments are not deployed more in the companies. I think that the technological performance of a system is a necessary condition to its deployment in the workplace, but it is not sufficient. I have thus identified three reasons to deploy AI which are not considered enough.
First of all, organisational acceptability. In other words, to what extent is the tool integrated within the organisation. Imagine an AI assisting a general practitioner in the identification of a skin disorder. The general practitioner acknowledges that the effectiveness of the system is first-rate, but the AI engenders an increase of consulting time to 30 minutes instead of the 15 usually allocated. The AI is here creating a problem of organisational acceptability and is not managing to fit into the organisation such as it is.
Then, social acceptability. To what extent will the system, even if it is effective, be refused or partially refused because it runs into difficulties with deep-seated values, constitutive of the profession. Let’s take the example of a tool which enables copies of pupils’ homework to be marked. On paper, this AI might seem to make teachers happy given that marking can ruin their weekends. But teachers do not necessarily see things that way. Because it is their responsibility to help the students to make progress, even if that involves marking hundreds of pieces of homework. The teachers are perhaps not ready to delegate this task. It would mean betraying the very essence of their profession. This is what Bourdieu termed the virile burden of work. There is a certain prestige in suffering at work, in being capable of putting up with long hours or repetitive tasks. If anybody could do this job, the cost of entry into a profession diminishes, and the individual cannot gain as much capital through their work. Their work thus loses value.
Finally, practical acceptability. To what extent can the AI system transform, abolish and/or create practices. Why is the question of practices important? Practices are areas of freedom at work and engage workers in their job. It is what creates their singularity. If an AI transforms, abolishes or creates a new practice, the world of work is turned upside down, as is the hierarchy of the worker in this world. Even if the new practices are not uninteresting, and even alleviate difficult working conditions, we see in the machine what de-singularises us and damages our professional identity.
Which are the jobs which, in your opinion, are the least likely to be impacted by AI?
Y. F.: At the moment, AI has made the most progress in non-repetitive high-level cognitive tasks. Quite the opposite of what was predicted about the future of AI regarding classical automation. It is in the end in cognition that it has progressed. All of the professions which enrol the body, and not the head or the mind, are thus a lot less exposed to AI. We are still light years away from being able to compare these humanoid robots to the tasks carried out by a human body. If we wish to globally automate a manual profession, such as driving, for example, we would have to rethink the entirety of the road infrastructure. For cognitive and abstract tasks, on the other hand, the constraints of the material world being slight, AI has made good progress.
What should be said to workers who remain resistant to AI? Should AI training courses be encouraged in the workplace?
Y. F.: First of all, we need to think of all the workers, and put behind us the dichotomy between subordinates, workers, employees, and on the other side paternalistic managers who insist on aiding their colleagues address the changes taking place even though they know no more than their workers. Why? Over time, the utilisation of AI on the basis of data requires not only more assistance but also a thinking through in terms of corporate culture. These empirical and learning machines are powerful and will progress further. If we retain procedural organisations in simply implementing a little bit of AI in their processes, without removing the layer of rationalisation, then we are heading for disaster. We mustn’t think simply in economic or productivist terms, but also at the level of the worker. Are the organisations and companies ready to cope with error, to adopt the ethos of AI based on data? The human being and the machine both make mistakes. But in workplace culture, error is still punished. However, if the error is also machinistic, it must be considered as part of a learning phase. For me, one of the fundamental tools remains social dialogue. We must stop developing AI whilst thinking that the workers know nothing about it anyway, so we can therefore implement it left, right and centre without explaining to them the approach behind it. The world of work does not need any more confrontation. It is vital that the entire ecosystem, from the suppliers of AI to the directors, including the social partners and the workers, everybody must be heard on this subject. At the present time, nobody is omniscient as regards AI. There is a need to create more collectives with a horizontal aim in order to move forward in the understanding of these intelligent systems.
Yann Ferguson is a Doctor of sociology at the National Digital Sciences and Technologies Research Institute (INRIA), visiting researcher at a French Research Center on Work Organizations and Policies (CERTOP) at the Université Jean Jaurès, and also the Director of Science at LaborIA, a research laboratory dedicated to AI and created by the Ministry of Labour, Full Employment and Social Integration and the INRIA in November 2021.
A story, projects or an idea to share?
Suggest your content on kingkong.