Choosing an AI model, the artists’ conundrum (2/2)
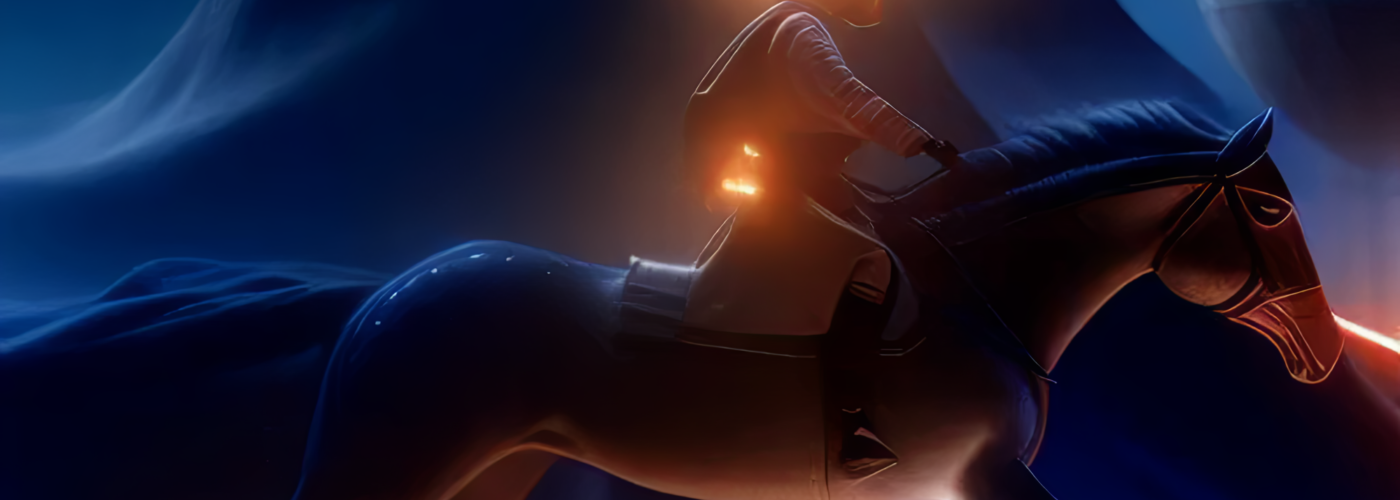
Article author :
The effects of the dataset
Despite the proliferation of generative artificial intelligence models, many artists still have a restricted view of the tools on the market and their impact. Having already looked into the issues of proprietary, free and open source models, this second part breaks down an essential point of these models: the dataset. Its makeup and the nature of the data have varied repercussions on the standardisation of aesthetics, on the environmental impacts or on the questions relative to copyright. An analysis based on expert testimonies.
This article is a republication. The original was published on HACNUMedia (the media that explores the connections between technology and creativity), a partner of kingkong.
Before getting into the crux of the subject, it is important to understand the utility of a set of data and revisit several technical aspects. A dataset consists of data (numbers, texts, images) and serves to train algorithms. Its main function is therefore to provide the algorithm with a diversity of examples in order for it to learn to recognise patterns, take decisions and make predictions. In other words, the dataset is indispensable for the AI systems which are the Large Language Models (LLMs) designed to process and generate text (ChatGPT, Google Gemini, LLaMA and Claude) or the image generators (Midjourney, Stable Diffusion, DALL-E). These models enabling texts or images to be generated are trained on enormous quantities of data found on websites and social networks (the most widely known technique is called web scraping), collected with the – more or less informed – consent of internet users. Let us first of all focus on the data used to train the LLMs and the image generators.
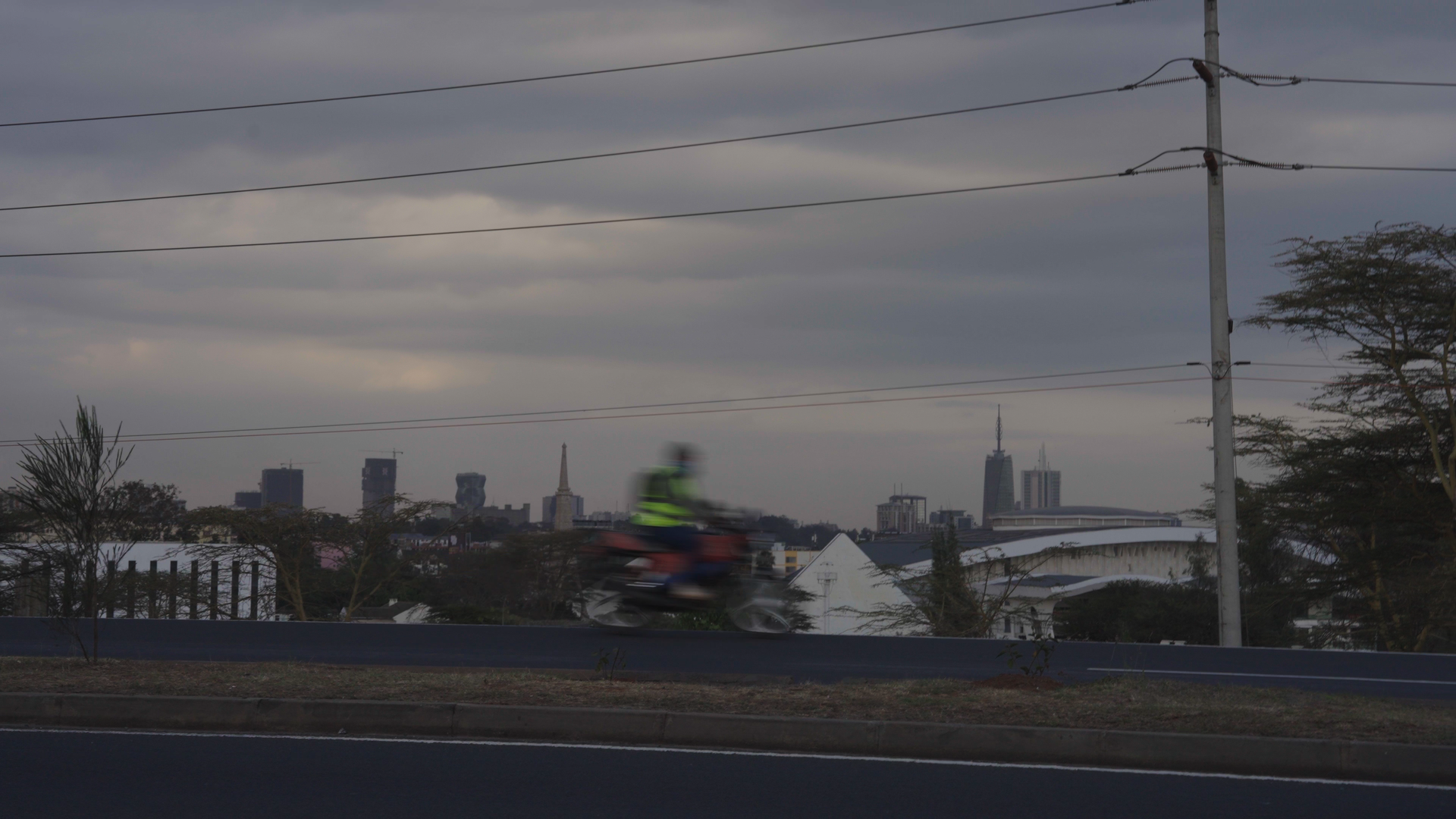
A mechanics of standardisation
This data, whilst substantial, remains generic: it to some extent forms a large scan of the internet and can lead to a standardisation of the responses generated. Conceptually, ‘with these models based on Big Data, you get a kind of photograph of the internet’s collective unconscious. It’s interesting, but the motor of creativity rests on constraint, for example on a restricted dataset,’ notes the artist Justine Emard, who has turned AI into the guiding thread of his work. Over time, this globalised dataset could have an unintended effect: the datasets gleaned from web scraping or web crawling (an indexing technique to automatically explore the Web) progressively include content supplied by generative AI models, thus contaminating existing models. That may trigger a cannibalisation of aesthetics effect. An hypothesis which is far from having been verified, given the way the LLMs continually evolve.
Likewise, the question of biases reinforcing racism or sexism, for example, is raised in the construction of these datasets. An aspect which should not be overlooked, but one which deserves being examined more deeply. The artist Grégory Chatonsky precisely shares his expertise in an article published on his website: ‘Statistical induction is criticized for its propensity to highlight certain points of view […] What is required in return? An absence of bias? Other biases? A transparency and a readability without any remnants of these biases? If AI is being fantastically transformed into an autonomous person with a personality, it should be viewed as a new way of browsing and consulting a library.’ To put it differently, let us not be blinded by subjectivity, nor naïve in this desire for objectivity and neutrality. ‘Criticism sets aside the historical hermeneutics inherent in all reading, and delegates even further to AI’s automatisms what should constitute our faculty of reflexivity. Criticism, as is often the case, reproduces what it believes it is contesting. By staging AI’s power of truth, it institutes it.’
Creating a made-to-measure dataset
Certain artists choose to build their own datasets in order to preserve their singularity, to cultivate their subjectivity. Ismaël Joffroy Chandoutis, an artist working at the intersection between contemporary art and cinema (read the article published on HACNUMedia) is an AI and deepfake specialist. He outlines several methods for integrating a dataset. For example, for an LLM, ‘it is possible to ‘create your dataset by pasting texts in the chat window. In this case, you must respect the word limit which the model can process, what is called the context-length token. If you need more, you can use a system which searches for additional information via RAG (retrieval-augmentation generation). That entails the building of an external database on a local or online server.’ Whilst the techniques for dataset creation differ again when it involves the generation of images, videos or sound, the issue remains crucial in every instance.
The artist Bruno Ribeiro, the author of several works produced by means of AI such as Polydactylie and CELLULOD/D, sees in this a way of being ‘independent and unique. My work MOTION (Editor’s note: presented at the Metahaus from October 18 to 25, 2024) which is an homage to the galloping horse of Eadweard Muybridge was produced on the basis of images from other films. I wanted it to be images which I knew, which I had chosen.’ While the machine enables one to go beyond what the eye cannot see, here the subjectivity of the artist remains at the centre of the approach adopted. Justine Emard shares his thought process during the creation of Hyperphantasia. For this work, an AI in Machine Learning (other than a LLM) was trained on a scientific database of the Chauvet Pont-d’Arc cave in order to manufacture new images. ‘I didn’t want to enter into a fantasy of prehistory. I wanted to stay within a form of abstraction. I therefore worked with the archaeologist Jean-Michel Geneste on a restricted dataset based on several thousand raw images. We selected and augmented them in an intelligent manner. With a generic database, the result would have been totally different.’
Although popular belief would have it that AI generates content instantaneously (and that it is therefore synonymous with saving time), it should be made clear that the building up of datasets is a long-term undertaking. ‘The establishing of datasets demands time. When you get started on the work you think it is quicker but everything takes longer,’ warns Bruno Ribeiro. And there are numerous stages: selecting the data, data pre-processing (cleaning), dividing the dataset into training data and test data (verifying the quality of the model), training, readjustment, assessment, improvement, etc. ‘The training takes several hours but the upstream and downstream phases can take months. It is also necessary to take the time to view hundreds of images created. It is a multi-layered process which is not instantaneous, unlike a prompt which generates an immediate image,’ testifies Justine Emard.
Environmental and social impacts
The dataset issue can also be examined from an environmental and social perspective. The LLMs rely on colossal datasets and require considerable computational power and resources. ‘This takes us back to the materiality of the digital. The data is stored on gigantic servers which need significant energy resources. The production of electricity, but also semi-conductors which entail the massive extraction of silicon and rare earths,’ explains Ismaël Joffroy Chandoutis. The data is treated by processors (GPU; Graphics Processing Units) carrying out AI calculations as well as video and graphics rendering. A model like ChatGPT makes use of several hundreds of thousands of GPUs for each training. Whilst no official announcement has been forthcoming, several sources estimate that the training of GPT-4o probably required at least 25,000 high performance GPUs over several months. ‘The NVIDIA H100 models are widely used today. Chips such as those contained in Neural Engine (Apple) look to optimise their impact by being more specialised and focusing solely on AI calculations. Despite everything, even though the environmental cost of these processors is being reduced, the levels remain astronomical,’ adds Ismaël Joffroy Chandoutis.
Otherwise, it is important to remind ourselves that numerous AI models, including those termed ‘unsupervised’, are calibrated thanks to human intervention. These ‘clickworkers’, often based in Madagascar or South-East Asia, are given the task of ‘annotating texts or images, in order to construct the learning corpus, for example by indicating on the photo of a crossroads which are the road signs, or by identifying the traces of rust on telegraph posts, or by noticing if a customer is in the act of stealing in a shop,’ explains the sociologist Clément Le Ludec in ‘Le Monde’. ‘Even what is termed generative AI is affected. ChatGPT required many annotations to teach the programme what an acceptable response is or isn’t, depending on a certain scale of values. In our database of companies making use of these human tasks, a third belong to the natural language processing sector.’ The work currently being created by Quentin Sombsthay, ‘Latente Image’ (2023 SCAM émergences Prize), precisely retraces the post-traumatic stress disorders experienced by these clickworkers based in Nairobi in Kenya. Paid 2$ per hour, they manually sort ultra-violent content in order to develop the censorship practiced by Chat GPT.
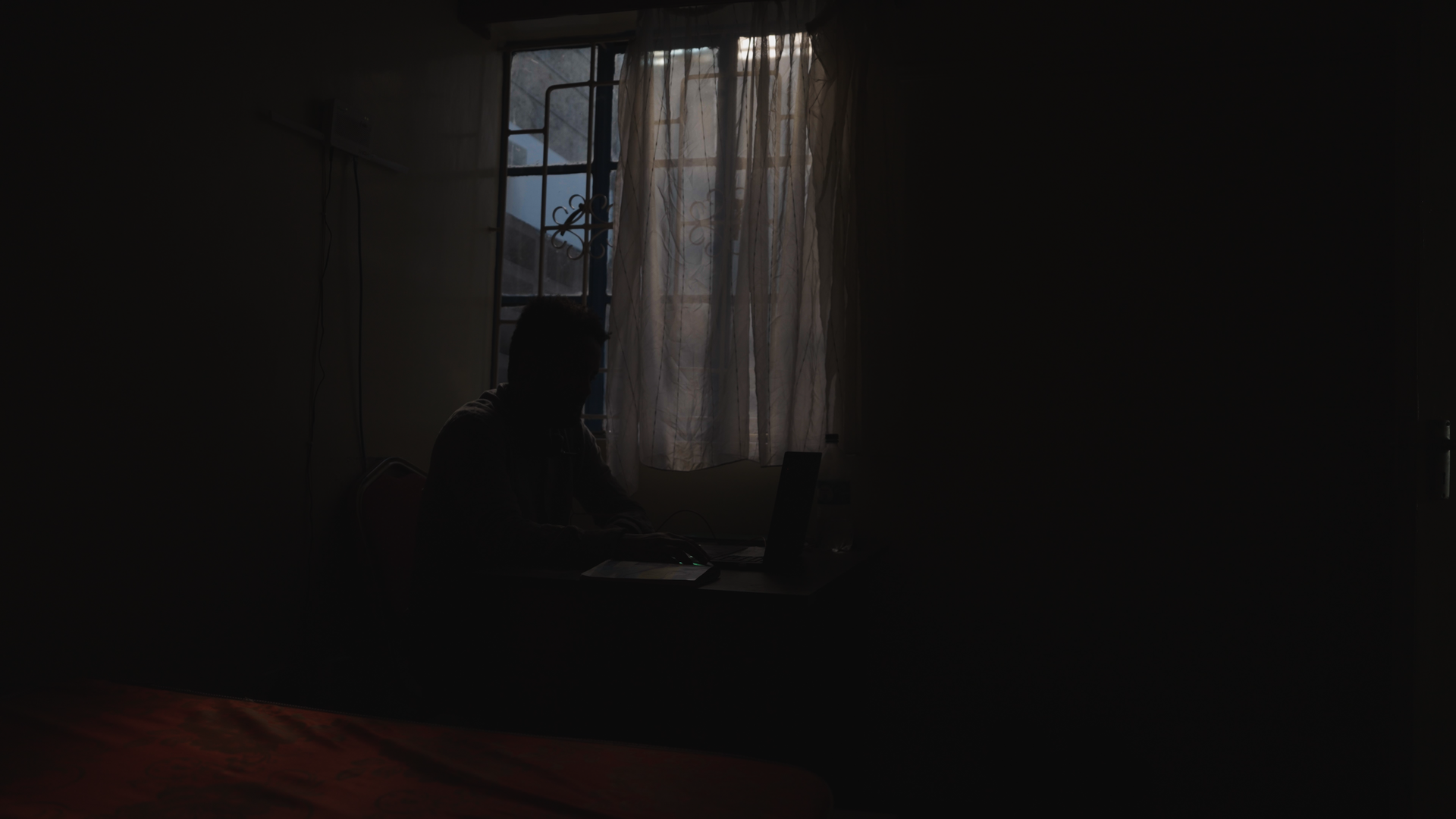
Training of data locally
Here again, the artists can minimise their environmental impact. ‘It’s an assessment each person has to make. There are compromises to be made between artistic coherence, financial resources and personal ethics. Personally, I wanted to work on a local server which redistributes the heat,’ shares Justine Emard. Training on local servers is on the other hand less compatible with the LLMs. ‘Technically, it’s possible, but that would require very powerful computers, which few people have access to, to process all of this data, otherwise the experience would be marred,’ points out Ismaël Joffroy Chandoutis. Hence the current enthusiasm in the world of Tech for SLM (Small Language Models). The difference between a Small Language Model and a Large Language Model principally lies in the size of their architecture, their computing capacity and, certainly, in the quantity of the training data. ‘We are progressively moving towards a cohabitation between the LLM and SLM models. For example, the strategy employed by Apple is to pivot their AI to the latest iPhones, in other words to local storage and with few watts,’ he adds.
The training of data can also be optimised (finetuning) via the des LoRAs (Low Rank Adaptation) technique which allows large size models to be finetuned. Nevertheless, it is worthwhile remembering that that the LLMs and the image generators are not the sole models on the market: there are other less advanced models based on Machine Learning, for example, and which may be all the better suited. Marc Chemillier, the Director of Studies at the Paris-based EHESS (School for Advanced Studies in Social Sciences), is the co-creator (together with other IRCAM (Institute for Research and Coordination in Acoustics/Music) researchers) of Djazz. ‘With the type of AI we use, the resources are very limited. Our model is not based on deep learning, it is a transition probability model. We can do impressive things with low quantities of data and little equipment. You just need to have a microphone and a computer. Then the software captures a musical flow and learns to play like it. It is an agnostic model without a particular rhythmic signature, just a regular beat which organises the data. The musical knowledge is in the flow we capture, then the AI creates an improvisation.’
Data subject to copyright
Finally, the sources of the data have been the subject of heated exchanges on the issue of copyright. Michal Seta, a creative technologist at Lab 148 in Montreal, sums up the debate: ‘there are several aspects to be analysed: the rights to the model (read the article published on HACNUMedia) but also the rights to the data which serves to train the AI and the protection of the production of a work generated by AI. The issue is one of knowing where the training data comes from. Models such as ChatGPT are trained on the basis of online media, Wikipedia, content published on social networks. These big companies are completely opaque concerning their dataset.’ In a similar vein, ‘the data sent in ChatGPT is used to train OpenAI. There is both a problem of confidentiality and one of consent.’ Recently an article published on HACNUMedia raised the question in these terms: ‘can the artists whose work has been provided to an AI be considered co-authors?’ If the recent publication of the AI ACT attempts to come up with responses, in particular with the obligation for these generative AIs to publish a detailed summary of the sources used for training, there is little room for manoeuvre. The anti-AI Watermarks (information subtly included within an image, a text or a video and used to protect creations against unauthorised use), or other projects such as the website HaveIBeenTrained which enable artists to have their images withdrawn from the databases of LLMs such as Stable Diffusion, are valuable but in the end have little impact. In this game of cat and mouse, the artists finally come up against an irrefutable reality: data is well and truly the black gold of the 21st century.
A story, projects or an idea to share?
Suggest your content on kingkong.
also discover
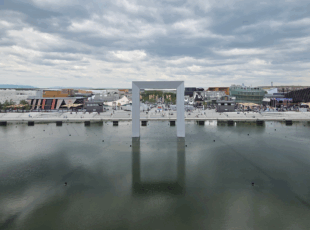
From Belgium to Japan, the new territories of creative digital creativity
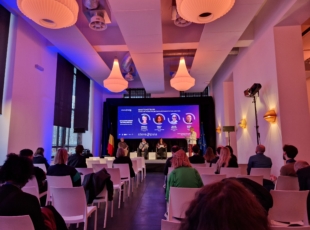
Stereopsia, the key European immersive technologies hub
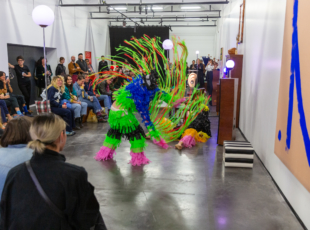
The Centre Wallonie-Bruxelles: a billion blue blistering harmonies
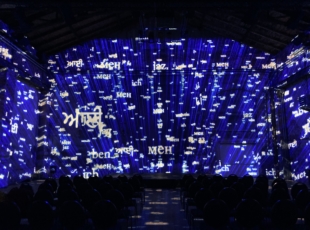